Boosting the power of single-cell analysis
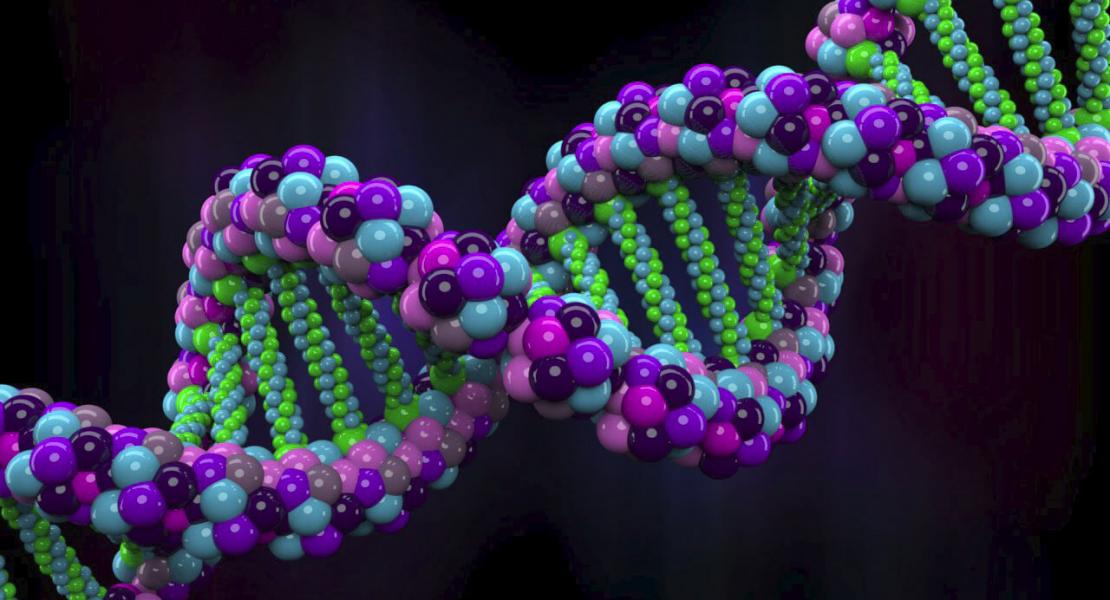
Abstract
Single-cell RNA sequencing (RNA-seq) is transforming our understanding of complex biological systems. Current technologies can measure the transcriptomes of thousands of individual cells in a single experiment, enabling the discovery of rare cell types and cellular heterogeneity that cannot be identified at the population level. However, combining data from different single-cell experiments is technically challenging, and without good tools, there is no way to fully harness the wealth of data that is being generated. In this issue, Haghverdi et al . [1] and Butler et al . [2] describe two computational approaches that correct for the technical errors, known as batch effects, that arise during meta-analysis of independent single-cell data sets. They show how these methods can uncover new biological variation and new cell types, suggesting the knowledge that can be gained by mining existing data sets.